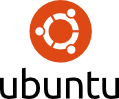
This is the default welcome page used to test the correct operation of the Apache2 server after installation on Ubuntu systems. It is based on the equivalent page on Debian, from which the Ubuntu Apache packaging is derived. If you can read this page, it means that the Apache HTTP server installed at this site is working properly. You should replace this file (located at /var/www/html/index.html) before continuing to operate your HTTP server.
If you are a normal user of this web site and don't know what this page is about, this probably means that the site is currently unavailable due to maintenance. If the problem persists, please contact the site's administrator.
Ubuntu's Apache2 default configuration is different from the upstream default configuration, and split into several files optimized for interaction with Ubuntu tools. The configuration system is fully documented in /usr/share/doc/apache2/README.Debian.gz. Refer to this for the full documentation. Documentation for the web server itself can be found by accessing the manual if the apache2-doc package was installed on this server.
The configuration layout for an Apache2 web server installation on Ubuntu systems is as follows:
/etc/apache2/ |-- apache2.conf | `-- ports.conf |-- mods-enabled | |-- *.load | `-- *.conf |-- conf-enabled | `-- *.conf |-- sites-enabled | `-- *.conf
- apache2.conf is the main configuration file. It puts the pieces together by including all remaining configuration files when starting up the web server.
- ports.conf is always included from the main configuration file. It is used to determine the listening ports for incoming connections, and this file can be customized anytime.
- Configuration files in the mods-enabled/, conf-enabled/ and sites-enabled/ directories contain particular configuration snippets which manage modules, global configuration fragments, or virtual host configurations, respectively.
- They are activated by symlinking available configuration files from their respective *-available/ counterparts. These should be managed by using our helpers a2enmod, a2dismod, a2ensite, a2dissite, and a2enconf, a2disconf . See their respective man pages for detailed information.
- The binary is called apache2. Due to the use of environment variables, in the default configuration, apache2 needs to be started/stopped with /etc/init.d/apache2 or apache2ctl. Calling /usr/bin/apache2 directly will not work with the default configuration.
By default, Ubuntu does not allow access through the web browser to any file apart of those located in /var/www, public_html directories (when enabled) and /usr/share (for web applications). If your site is using a web document root located elsewhere (such as in /srv) you may need to whitelist your document root directory in /etc/apache2/apache2.conf.
The default Ubuntu document root is /var/www/html. You can make your own virtual hosts under /var/www. This is different to previous releases which provides better security out of the box.
Please use the ubuntu-bug tool to report bugs in the Apache2 package with Ubuntu. However, check existing bug reports before reporting a new bug.
Please report bugs specific to modules (such as PHP and others) to respective packages, not to the web server itself.